I have been taking photos, screenshots from my computer, printing them, re-photographing them, cropping them on my phone and reprinting them, creating assemblages that follow connections and human neural networks. In this process I take the role of a generative AI, but the images I generate are assemblies of layered meaning, sometimes obtuse, sometimes open. Coppélia, 2023, is an example of these assemblies of layered meaning, whereby my friend’s long eyelashes become part of the cover of Léo Delibes’ Coppélia (1870) and a screenshot of the famous menacing look from Stanley Kubrick’s Clockwork Orange (1971). Behind the Clockwork Orange image, a pdf of one of Spinoza’s book peeks through. As I create these assemblages, I have an open dialogue with an imaginary machine learning model. I wonder what it sees, what connections it can make, what kind of imitation, apprenticeship, or transfer learning model I need to create to insert the emotional, sensual, and animated and layered information that is captured in these assemblages. In Coppélia, the meaning of all 3 images is altered as they enter in conversation with each other and the shadow of the images as they are taped on the wall adds an additional texturality and dimensionality to the work, see figure 1.
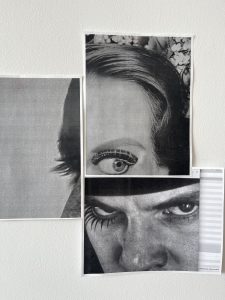
By exploring the surface of the wall as an infinite canvas where I can encounter and converse with these images in a dynamic and lived manner, I participate in Giuliana Bruno’s (2016) view of surface encounters as spaces, where “novel dynamics are generated” and a new form of materiality emerges that is open, malleable and “permeable.” These surface encounters take place between the images as they are taped on the wall, but also within them. The contextual information nested in them, as the virtual and physical edges of each animates the other; an aural dimension enters their inert surface, the material resonance of the paper, as each photographic paper carries its own frequency, the play of light and shadow on the wall – they all participate in a genre of animated surface that points to an alternativeview of what multimodality in machine learning models can mean. While the think tank Antikythera states that in multimodal large language model (LLM) interfaces “the design space of interaction with multimodal LLMs is not limited to individual or group chat interfaces, but can include a diverse range of media inputs that can be combined to produce a diverse range of hybrid outputs,” I am interested in exploring this multimodality as a property that can be contained within the image assemblages themselves.
Progressing from Katherine Hayles (2017) writing on “unthought,” the unthought in this series of works emerges from the use of my technological tools (my phone, laptop, printers), which become extensions of my memory and emotional connective tissue, and the speedy composition of these assemblages. Part journal, part memory aide, part an attempt to bring materiality to images as they become increasingly virtual, and an attempt to collaborate and corroborate with artificial neural networks by experimenting with my own neural network as I converse with the various machines and machinic models around me. I create images as training data sets, part of a machine learning (ML) model that looks at the emotional and narrative connections, and their material implications. In the tokenized space between assemblages and model, I insert a sense of affect in the form of emotion vectors to machine learning models, see figure 2. In both the composition of the image assemblages and in the development of the machine learning model, I think of Donna Haraway’s Companion Species Manifesto (2003), whereby she writes about a relation to technology as a companion in which an emergent sense of affinity between machine and human, a sense of co-evolution and companionship that gives form to a hybrid embodiment to both human and machine. Much like animated characters that morph in and out of frame.
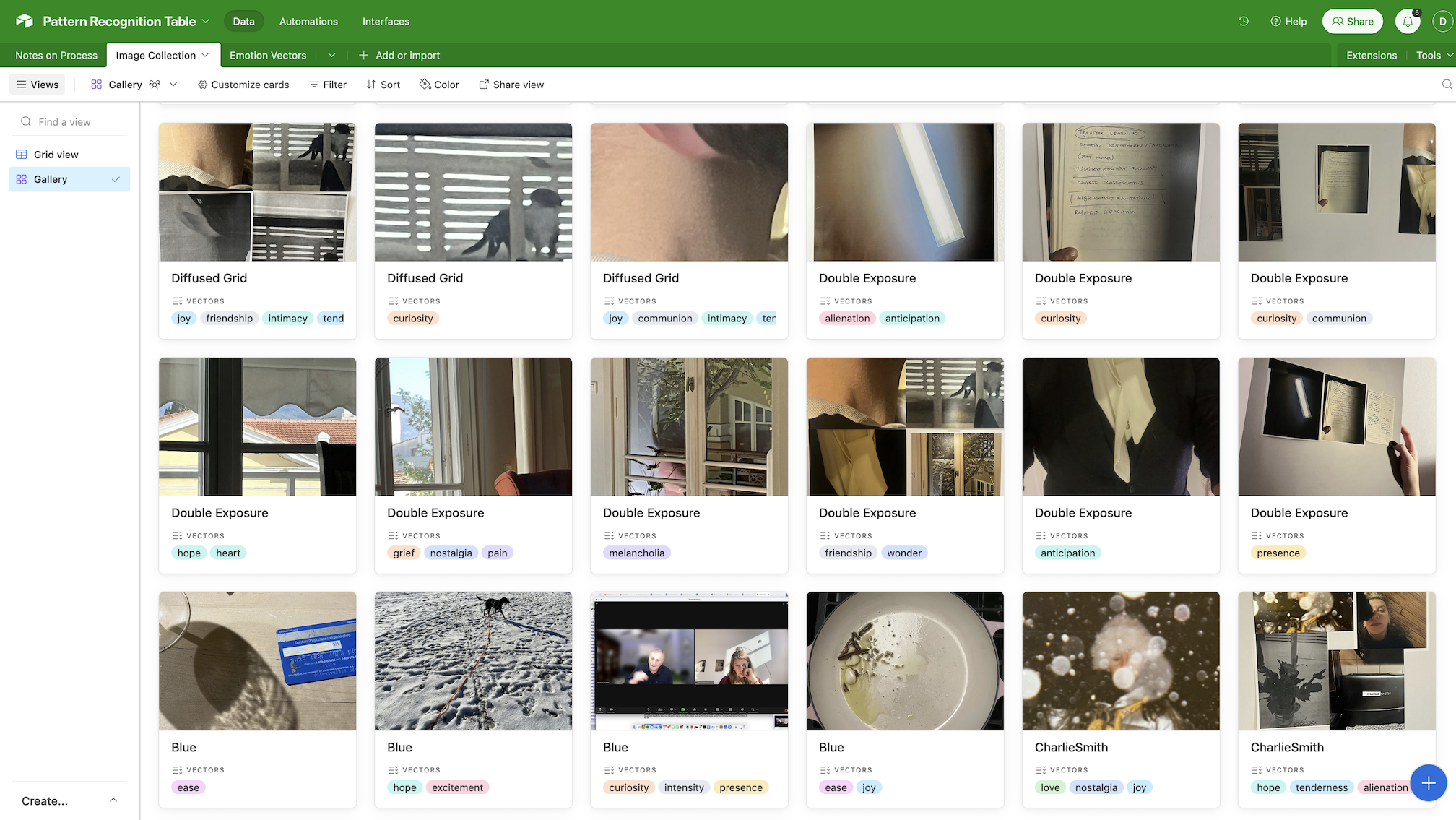
Such a process questions the limits of what can be communicated to machine learning models in its attempt to re-insert the anima, the emotion, affect, and resonance, both to the production and consumption of such models. Here, I take Norman McLaren’s definition of animation as quoted in Maureen Furniss (2007, p. 5), where:
Animation is not the art of drawings that move but the art of movements that are drawn. What happens between each frame is much more important than what exists on each frame. Animation is therefore the art of manipulating the invisible interstices that lie between the frames.
Norman McLaren
This understanding of anima and animation, brings forth the a relationship to emotion and affect that introduces complexity, nuance and multi-dimensionality in interactions with machine learning models. This potentially also contributes towards an expanded vocabulary and emotional narrative structures for generative AI systems, in so far that Cheng Li et al. (2023) suggests that “emotional prompts” can optimize the performance of large language models (LLM), arguing that the ability to comprehend and respond to emotional stimuli is a crucial characteristic of the human problem-solving ability.
In his definition of affect, philosopher Spinoza (2002) firmly grounds emotion in a dynamic interplay between body, mind and environment, stating that “by emotion [affectus] I understand the affections of the body by which the body’s power of activity is increased or diminished, assisted or checked, together with the ideas of these affections” (p. 278). This interplay of a dynamic relationship between bodies, spaces, ideas and images offers an expanded understanding of what the role of animation and its relationship to the animated and in-animate can be, especially as images start to be animated void of emotion or context in generative AI systems. This understanding has the potential to alter relationships to technology, and therefore to space, time, motion, emotion, and stillness.
References
Antikythera. Incubated by Berggruen Institute, accessed February 17, 2024, https://antikythera.org/synthetic-intelligence.
Bruno, Giuliana. Surface: Matters of Aesthetics, Materiality, and Media. Paperback edition. Chicago London: University of Chicago Press, 2016.
Furniss, Maureen. Art in Motion: Animation Aesthetics. Rev. ed. Eastleigh, UK : Bloomington, IN: John Libbey ; Distributed in North America by Indiana University Press, 2007.
Haraway, Donna. The Companion Species Manifesto: Dogs, People, and Significant Otherness. Paradigm 8. Chicago: Prickly Paradigm Press, 2003.
Hayles, N. Katherine. Unthought: The Power of the Cognitive Nonconscious. Chicago ; London: The University of Chicago Press, 2017.
Li, Cheng, Jindong Wang, Yixuan Zhang, Kaijie Zhu, Wenxin Hou, Jianxun Lian, Fang Luo, Qiang Yang, and Xing Xie. “Large Language Models Understand and Can Be Enhanced by Emotional Stimuli.” arXiv, November 12, 2023. http://arxiv.org/abs/2307.11760.
Spinoza, Benedictus de. Complete Works. Edited by Michael L. Morgan. Translated by Samuel Shirley. Indianapolis, IN: Hackett Publishing Company, Inc, 2002.
Despina Papadopoulos is an artist working at the intersection of technology, philosophy and embodied systems. She is a PhD candidate at the School of Arts and Humanities at Royal College of Art and a Visiting Professor at the Collaborative Arts Program at Tisch School of the Arts, NYU. Her current research is concerned with philosophical theories of machine learning systems, exploring ways to insert plurality, the logic of sense and variability in mechanic infrastructures.