The connection between AI and animation is currently widely discussed with regard to the influence of machine learning technologies on animation production (e.g., see The Next Leap: How A.I. will change the 3D industry – Andrew Price). What animation is or can do therefore seems to be more and more determined by AI (which brings up additional questions regarding the agency of the animator and the state of creative labor and so on). What remains mostly neglected in this perspective, however, is that there is also a ‘reverse’ (or, more precisely, interdependent) relationship between animation and AI, in which not only animation is negotiated and (re)defined through the use of AI techniques, but conversely, the determination of what AI actually is and can do seems highly dependent on how it appears in animated images.[1] Thus, the epistemic question I will touch on very briefly in this blog post is not: “To what extent is animation – in its technological realization – currently defined by AI?”. I will vice versa touch on the question: “To what extent is AI – as a real technology – currently defined by its appearance in animation?” Or to put it differently: In how far is animation a documentary medium for AI? To pursue these questions, I would like to look at a concrete example that might help to illustrate this reciprocal relationship between AI and animation.
Documenting “the mind of a hallucinating robot brain”. In 2019, Jon Warlick (a wedding videographer from North Carolina) uploaded a video called “Generating Photorealistic Video with AI Part 1” to YouTube[2]. In this video Warlick describes and shows his experiments with NVIDIA’s AI image generator GauGAN, a Generative Adversarial Network that produces attempted photorealistic images based on rough digital sketches (with an interface reminding of MS paint; see Fig. 1). But as GauGAN generates only single images – one at a time – this does not seem to satisfy Warlick’s attempt to explore the creative and technological capabilities of AI. “How can I create an animated video from the mind of a hallucinating robot brain?”, he wonders in the video’s voice-over and then starts to develop a workflow that allows him to turn GauGAN’s single frame outputs into animation.
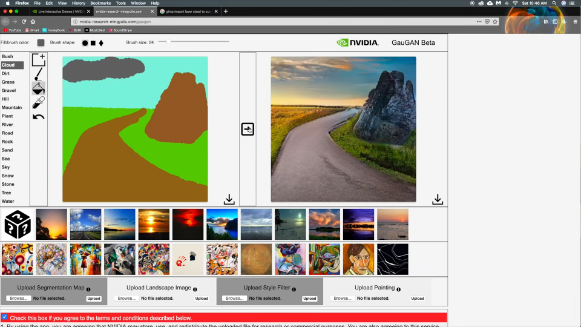
To achieve that, Warlick first creates a landscape painting in GIMP, using the identical color palette of the GauGAN toolset, and then records a movement through this landscape with the help of After Effects (first in a 2D, then in a 3D version) (see Figg. 2 and 3).
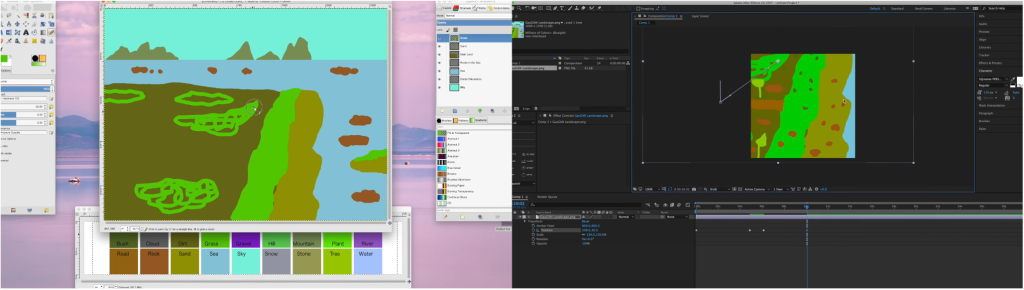
As GauGAN does not allow to upload video files, he has to export the finished video sequence as single-frame images, import them one by one into GauGAN to submit them to the AI mechanism, and afterwards reassemble them into a video sequence again. “I finally had all my frames generated and it was time to see what a robot thinks the world looks like” – Warlick summarizes the last step of his endeavour. The outcome is a fascinating ‘glitchy’ and flickering video sequence of a (not really) photorealistic landscape that seems to fail in any attempt to generate a homogenous, believable impression of a world ‘as we know it’ (see video below). But this is exactly the point: as these images reveal themselves as strange, alienated, and deviating from ‘what we know’, they seem to unveil ‘what the machine knows’. The “robot brain”, so to say, becomes visible in the clashes of the frames.
Intelligence in between – Processuality, Seriality, and Transformation in AI Animation. What I find so interesting about this video with regards to the relation of animation and AI is that it addresses their interlinkage on a (techno)logical as well as aesthetic level. Why, one could ask, does Warlick feel the urge to produce an animated film out of GauGAN’s single images? The answer might be that he was just bored or interested in a new filter aesthetic for his wedding videos; but I think another reason might be more convincing: Something seems to be missing in the single frames that comes “to life” in the animated sequence. To generate an impression of what artificial intelligence and computer vision is (or at least looks like – in an active as well as passive sense), it seems to be necessary to show it in a moving image sequence and not in stills.
Three aspects are relevant in this attempt to make artificial intelligence visible – and thus to document it: processuality, seriality, and transformation.[3]
The AI mechanism in this process is presented as a tool that translates a rudimentary digital ‘painting’ into something that it ‘thinks’ looks like ‘the outer world’. Thus, the transformation of the image can be taken as proof and illustration of this thinking process. But while this translation might become apparent in the comparison of two single frames (like in GauGAN itself; see Fig. 1), it becomes even more evident and illustrative when this operation is repeated over and over again. The GauGAN interface already supports this idea of the relevance of image seriality as it offers different styles and interactions with the material and thus encourages the user to produce not only one but multiple images.[4] To get an idea of the machine’s thinking thus implies to experience it as a process[5] – and this is where the animation as a documentary tool[6] comes into play: The process of thinking becomes perceivable in the processuality and seriality of the animated images, in the differences and transformations between the single frames that are revealed in the flickering and glitchy aesthetics of the video. In short: the intelligence is what happens ‘in between’ the frames.
That AI is fundamentally based on processes of image comparison becomes even more striking when Warlick uses a split-screen to show the original video file he created in GIMP and After Effects next to the AI transformed version of the clip (see Figg. 4 and 5).[7] The intelligence of the machine reveals itself in these aesthetics of comparison and difference that not only derives from the ‘glitchy’ look of the video itself but from its juxtaposition to the PAINT-like animated source material as well. The AI becomes visible as a (special) image effect by the comparison of different styles of animation – abstract, reduced and ‘poor’[8] on the one side vs. attempting ‘photorealistic’ or photo-mimetic, but nevertheless abstract in its glitches on the other.
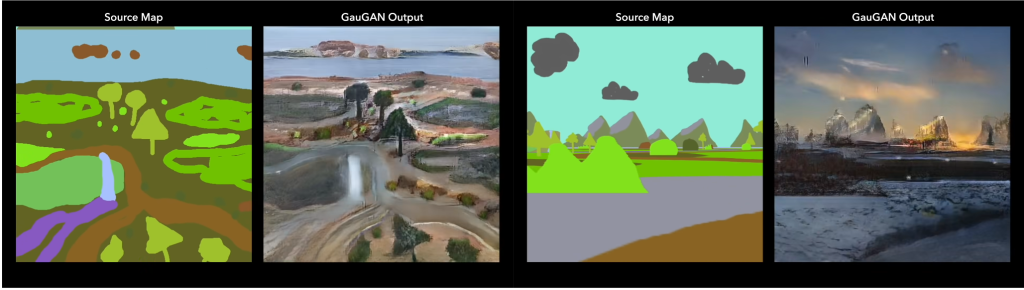
Indexing Intelligence – AI as a ‘Special Effect’ of the (Digital) Real. The main argument of this post is therefore that animation appears to be the ideal filmic art form that allows for the documentation of Artificial Intelligence, as both are based on the logics of single frames that generate their effect only through their combination and transformation in a series of images. Or to put it differently: The fundamental asset of animation, to be an art form that is based on the aesthetics of frame-by-frame image succession and differentiation, allows for AI to reveal itself as an art(ificial) form that bases its intelligence on exactly this mechanism as well.
Referring to Nea Ehrlich’s recent book on Animating Truth (2021), this comes as no surprise: In the “mixed realities” of contemporary digital culture, as she writes, animation is the aesthetic realm in which this culture can be adequately documented. Therefore, animation becomes an index of the digital in a broader sense (Ehrlich 2020) – with Artificial Intelligence being part of it.
On a methodological level, this means to have a look at materials (like the one mentioned above) in which AI is negotiated in and through animation: Tech demos, how-to-videos, deep fake breakdowns, making-ofs or other tech-oriented audiovisual formats[9] that deal with and discuss the application of AI therefore promise to reveal it as a special effect of the (digital) real in which the attempted intelligence of the machine leaves its traces between the frames to be documented.
References
Eckel, Julia. 2021. “Tech Demo/Tech Doku. Zur Wirklichkeit des Animierens“. In In Wirklichkeit Animation … edited by Franziska Bruckner, Juergen Hagler, Holger Lang, Maike Sarah Reinerth, 11–32. Wiesbaden: Springer VS.
Ehrlich, Nea. 2021. Animating Truth. Documentary and Visual Culture in the 21st Century. Edinburgh: Edinburgh University Press.
Ehrlich, Nea. 2020. “The Animated Document: Animation’s Dual Indexicality in Mixed Realities.” Animation: an interdisciplinary journal 15, no. 3 (November 2020): 260–75. https://doi.org/10.1177/1746847720974971.
Honess Roe, Annabelle. 2013. Animated Documentary. London: Palgrave Macmillan.
Murray, Jonathan, and Nea Ehrlich (eds). 2018. Drawn From Life. Issues and Themes in Animated Documentary Cinema. Edinburgh: Edinburgh University Press.
Steyerl, Hito. 2009. “In Defense of the Poor Image.” eflux journal 10 (Nov 2009).
Torre, Dan. 2017. Animation – Process, Cognition and Actuality. London: Bloomsbury.
Jun.-Prof. Dr. Julia Eckel is Junior Professor for Film Studies at the Department of Media Studies at Paderborn University, Germany. Currently, she is working on a book on the nexus of animation, documentation, and demonstration, but she is also interested in audiovisual anthropomorphism, synthespians, animation and agency, screencasting, and selfies. Recent publications include Das Audioviduum. Eine Theoriegeschichte des Menschenmotivs in audiovisuellen Medien (2021) and the two co-edited volumes Ästhetik des Gemachten. Interdisziplinäre Beiträge zur Animations- und Comicforschung (2018) and Im Wandel … Metamorphosen der Animation (2017). For more info see: www.juliaeckel.de.
[1] See also the blog post published next week here on Animation Studies 2.0 by Nea Ehrlich and me on this topic.
[2] The video is followed by a second part that presents an automated process for the single frame input/output routine in GauGAN (see Generating Photorealistic Video with AI Part 2). Additionally, Warlick’s channel offers several music videos produced based on the developed image generation procedure.
[3] For further considerations on the topic of seriality and the shared frame-by-frame logics of AI and animation, I again refer to the upcoming post by Nea Ehrlich and me on this blog.
[4] While at the same time, the Machine Learning itself is based on the analysis of seemingly endless series of similar but different images compiled in training data sets.
[5] It would be very fruitful to think about this processuality a bit more thoroughly, for example with regards to Dan Torres book on Animation – Process, Cognition and Actuality (2017).
[6] This idea could be further embedded in broader debates about documentary animation, of course (see e.g. Honess Roe 2013; Murray; Ehrlich 2018; Ehrlich 2021).
[7] Warlick uses this comparison in several of his other ‘AI generated videos’ as well to enhance the “Wow”-effect it seems (that AI can create these “trippy” landscapes from most simple sketches).
[8] Steyerl’s idea of the “poor image” in its relation to truth becomes interesting here as well (Steyerl 2009).
[9] Regarding the nexus of animation and documentation in formats like “Tech-Demos” and “Tech-Dokus”, see also Eckel (2021).
1 comment for “Intelligence In Between: Documenting AI in Animation”